Keynote Speakers
Keynote 1
– Place : #301, 3F
– Session Chair : Prof. Flavius Frasincar (Erasmus Univ. Rotterdam, the Netherlands)
Prof. Bing Liu (University of Illinois at Chicago, USA)
“Lifelong Learning for Sentiment Analysis” Download
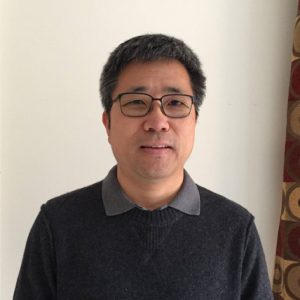
Short bio
Bing Liu is a distinguished professor of Computer Science at the University of Illinois at Chicago (UIC). He received his Ph.D. in Artificial Intelligence (AI) from the University of Edinburgh. Before joining UIC, he was a faculty member at the School of Computing, National University of Singapore (NUS). His research interests include sentiment analysis, lifelong learning, natural language processing (NLP), data mining, machine learning, and AI. He has published extensively in top conferences and journals. Two of his papers have received Test-of-Time awards from SIGKDD (ACM Special Interest Group on Knowledge Discovery and Data Mining). He is also a recipient of ACM SIGKDD Innovation Award (the most prestigious technical award from SIGKDD). He has also authored four books: two on sentiment analysis, one on lifelong learning, and one on Web mining. Some of his work has been widely reported in the international press, including a front-page article in the New York Times. On professional services, he served as the Chair of ACM SIGKDD from 2013- 2017, as program chair of many leading data mining conferences, including KDD, ICDM, CIKM, WSDM, SDM, and PAKDD, as associate editor of leading journals such as TKDE, TWEB, DMKD and TKDD, and as area chair or senior PC member of numerous NLP, AI, Web, and data mining conferences. He is a Fellow of the ACM, AAAI, and IEEE.
The classic machine learning (ML) paradigm works in isolation and makes the closed-world assumption. Given a dataset, a ML algorithm is executed on the data to produce a model. The algorithm does not consider any other information in model training and the trained model cannot handle any unexpected situations in testing or applications. Although this paradigm has been very successful, it requires a large amount of training data, and is only suitable for well-defined, static and narrow domains. In contrast, we humans learn quite differently. We always learn with the help of our prior knowledge. We learn continuously, accumulate the knowledge learned in the past, and use it to help future learning and problem solving. When faced with an unfamiliar situation in an open environment, we adapt our knowledge to deal with the situation and learn from it. Lifelong learning aims to achieve this capability. In this talk, I will give an introduction to lifelong learning and discuss some of its applications in sentiment analysis and beyond.
Keynote2
– Place : #301, 3F
– Session Chair : Prof. In-Young Ko (KAIST, South Korea)
Prof. Sunghun Kim (NAVER Corp., South Korea / Hong Kong University of Science and Technology, Hong Kong)
“Managing Deep Learning Debt @Naver/Line”
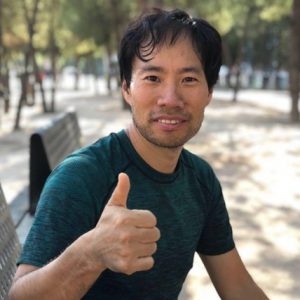
Short bio
Sunghun Kim is an Associate Professor of Computer Science at the Hong Kong University of Science and Technology, and currently leading the Naver Clova AI team. He got his BS in Electrical Engineering at Daegu University, Korea in 1996. He completed his Ph.D. in the Computer Science Department at the University of California, Santa Cruz in 2006. He was a postdoctoral associate at Massachusetts Institute of Technology and a member of the Program Analysis Group. He was a Chief Technical Officer (CTO), and led a 25-person team at the Nara Vision Co. Ltd, a leading Internet software company in Korea for six years. His core research area is Software Engineering, focusing on software evolution, program analysis, and empirical studies. He publishes his work on top venues such as TSE, ICSE, FSE, AAAI, SOSP, and ISSTA. He received the Most Influential Paper Award at ICSME2018 (from his ICSME 2008 paper). He is a four-time winner of the ACM SIGSOFT Distinguished Paper Award (ICSE 2007, ASE 2012, ICSE 2013 and ISSTA 2014). He served on a variety of program committees including ICSE, FSE, and ASE. He was a program co-chair of MSR 2013 and 2014. He is the general chair of MSR 2020. He is an associate editor of IEEE Transactions on Software Engineering and Empirical Software Engineering. His online deep learning course, https://www.youtube.com/user/hunkims has more than 4M views and 30K subscribers. Further information is available at http://www.cse.ust.hk/~hunkim
Deep learning has shown amazing results in both academic and industry, yielding many practical services and products such as machine translation, personal assistant, image retrieval, video recommendation and photo enhancement. However, in industry, it is common to observe legacy products with deep learning debt – the tremendous cost in converting traditional machine learning systems to deep learning. In order to utilize deep learning and boost the performance of existing products, it is necessary to examine our systems and repay any deep learning dept. This talk will describe my experience at Naver/LINE on managing deep learning dept, including challenges and their potential solutions.
Keynote 3
– Date & Time : Friday, June 14th, 09:00-10:30
– Place : #301, 3F
– Session Chair : Prof. Maxim Bakaev (Novosibirsk State Technical Univ., Russia)
Prof. Antti Oulasvirta (Aalto University, Finland)
“Artificial Intelligence and the User Interface” Download
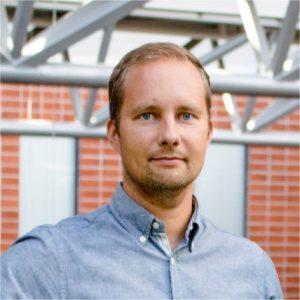
Short bio
Antti Oulasvirta leads the User Interfaces research group at Aalto University. He was previously a Senior Researcher at the Max Planck Institute for Informatics and the Cluster of Excellence on Multimodal Computing and Interaction at Saarland university. He received his doctorate in Cognitive Science from the University of Helsinki in 2006, after which he was a Fulbright Scholar at the School of Information in University of California-Berkeley in 2007- 2008 and a Senior Researcher at Helsinki Institute for Information Technology HIIT in 2008- 2011. He was awarded the ERC Starting Grant (2015-2020) for research on computational design of user interfaces. His work has been awarded the Best Paper Award and Best Paper Honorable Mention at CHI twelve times between 2008 and 2018. He has held keynote talks on computational user interface design at NordiCHI’14, CoDIT’14, EICS’16, and IHCI’17.
This talk describes on-going work towards intelligent user interfaces that better understand users and can better adapt to them. I discuss a type of machine intelligence where causal, predictive models of human-computer interaction are used with probabilistic inference and optimization. Several examples are presented from graphical user interfaces to the web. Keywords: Intelligent user interfaces · Machine intelligence · Computational modelling · Probabilistic inference · Combinatorial optimization.